Why Innovation?
The pace of change can be overwhelming for innovation teams. But, we can’t stand still or we will be outpaced in the marketplace. We’re driven to innovate not just to stay apace with the competition, but because creativity is an essential part of human nature. It drives our search for “new strategies to diagnose, treat, and prevent diseases.”
Generative AI is emerging as a powerful accelerant to the innovation process. It can improve your process in three key ways: capacity, speed, and quality.
A growing body of research, shows that it delivers impact across the innovation process: "Generative AI may be a game changer..., as the delegation of tasks to an artificial agent can result in faster iterations and reduced costs." The result is a new "transformative phase in which individuals and organizations increasingly turn to ai systems…"
Indeed, a May 2024 survey by McKinsey found that 65% of companies are using Generative AI in “at least one business function.” A full 23% are using GenAI in product or service development, and 10% listed innovation as their most common use-case.
Innovation Process
Creativity is the process of generating new, novel ideas. Innovation is the process of successfully implementing those novel ideas within your organization. AI and creativity can be fused to enhance innovation! Common methodologies, including Design Thinking, TRIZ, or Double Diamond, all follow a common pattern:
- Problem identification - where the team recognizes gaps and clearly articulates problem statements, goals and objectives for solving them.
- Research and ideation - the team gathers and analyzes data relating to the problem space, and generates potential ideas.
- Concept development and testing - the ideas are refined and tested in an iterative fashion.
- Implementation - planning and launch of the selected idea, performance monitoring and scaling.
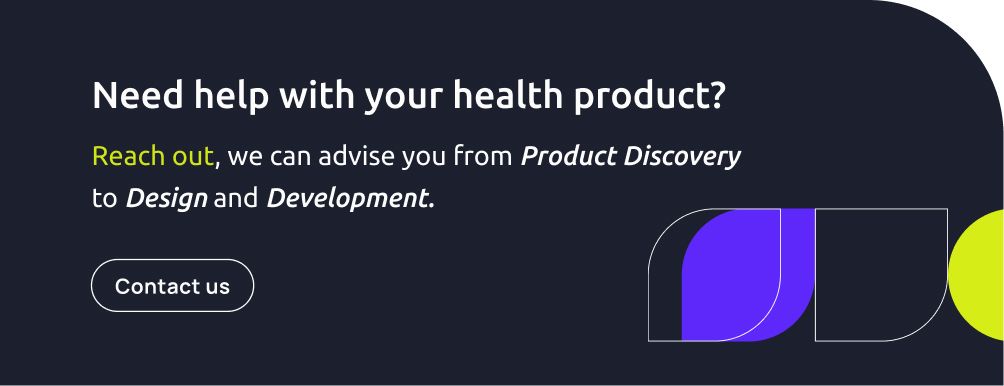
Generative AI can be applied at all stages of the innovation process. You can use it to explore the problem space to identify trends and reveal insights. Ideation and concept evaluation are fertile grounds for GenAI. Indeed, it may even already "outrival human idea generation regarding overall idea quality.”
And GenAI can greatly speed up prototyping - enabling your team to rapidly generate high-fidelity concepts, mockups, and code. It has even been used to design a new microchip, where “... the language model seems to be a ‘force multiplier’, allowing for rapid design space exploration and iteration” in the development of a novel processor.
The Double Diamond is a common innovation framework, with successive expansion and narrowing of focus. Using GenAI essentially expands the Double Diamond across a broader problem and solution space, facilitating the generation, consideration, and development of additional ideas or concepts.
Problem Identification
The first stage of the innovation process is selecting and articulating the problem to address. Indeed, many innovation activities fail because they attempt to solve the wrong problem. Leveraging AI for sentiment analysis of customer data at scale can give the team a clearer understanding of your customer’ needs at scale.
Ideation
During Ideation, you can prompt the AI system to review a broader scope of knowledge collected during the exploration phase, and use it to generate novel concepts that align to “a particular context, user segment, or user needs.”
To assess which ideas are worth developing, innovation teams often score or rank their ideas to facilitate filtering out the less promising ones. One methodology is to score them on Novelty, Benefit to the Customer, and Feasibility. You can then calculate a Quality score by multiplying those scores, so Q=(N*B*F).
And the stronger you can drive alignment and a shared mental model of the vision from the outset, the closer to it your outcomes will be.
Concept Development
Research shows that when we invite Generative AI into our process and treat “algorithms rather as members of an innovation team,” as opposed to a replacement for it, we improve the quality of our concepts, and radically increase the pace of innovation. It helps us avoid focusing on the wrong problem. Indeed, alignment around problem selection is one of the biggest challenges in innovation. "Augmenting human innovation teams with AI hence calls for investigating many aspects of jointly working with AI rather than only human colleagues."
Jan Joosten, and his team at the BeLab-Behavioral Studies and User Experience Laboratory in Germany, ran a blind test to compare the quality of ideas generated by AI with those generated by professionals.
They recruited a European packaging company, and assigned them two tasks: design new packaging solutions that added value to their customers, and design new packaging solutions that aligned with their ESG goals. The researchers also prompted ChatGPT 3.5 with the same tasks. The human designers generated 43 concepts and the GPT generated 52. The researchers were then graded by the company’s director of innovation in a randomized order. In this study, humans scored lower in both novelty (0.0039) and customer benefit (0.0028) with a negligible difference in feasibility (0.2922). Overall quality score: 0.0039.
Implementation
But that’s not the end of the story. When humans and AI work together, we get better results than humans or AI alone. By using AI, we can “add an entirely new capability” to our teams. This new capability “puts nontechnical users in a position to close the gap between conceptual work (i.e., ideas and concepts) and early look-and feel-like prototypes that can be tested with users.”
And AI can be applied along the entire innovation pipeline. When defining the problem, you can use it to quickly extract knowledge from a vast amount of data.
You can perform sentiment analysis with it to generate unique insights. Once you’ve identified your problem, you can then augment your ideation with AI. Again, another space where humans and AI together can form “superminds” such as in Prediction Markets. And then it can help you create prototypes more quickly and efficiently. AI in innovation is a game-changer!
AI Collaboration Strategies
When collaborating with Generative AI, there are several strategies you can use to get the best results.
1. Use the CARE framework for your prompt: Provide the background context, Explain the action you want the LLM to take, Your expected result, and a clear example:
- Content: We need to optimize our administrative processes to reduce patient wait times and paperwork.
- Action: Propose solutions for automating and digitizing administrative tasks.
- Result: Efficient workflows that minimize manual input and streamline patient check-in and record-keeping.
- Example: Introducing a self-service kiosk system where patients can check in and update their information without needing to fill out paper forms.
2. Few-shot - this technique includes several examples of the results you want in your prompt - to give the LLM a clear picture of your desired outcomes.
3. Chain of thought - Asking the LLM to explain the process is another technique that can lead to better results, rather than leaping straight to the output.
4. LLMs excel at interpreting natural language prompts, but that doesn't mean you can’t use structured data as well. You can use Markdown Tables or JSON both in your input and your output from the AI.
5. When you find a prompt or workflow that works, share it with your team. Create a prompt library, or create a custom GPT to increase efficiencies and knowledge sharing.
Reduce Risk with Humans in the Loop
Benefits aside, there are still legitimate barriers to wholesale adoption. Due to the “black box” of their operation, it can be hard to really trust the output of a LLM. If we don’t have transparency around what data the model was trained on, we can miss bias in the output, or encounter hallucinations. But that is starting to change.
Researchers at Anthropic released a paper describing the first “mapping” of the patterns of neuron activations inside the model directly to human-interpretable concepts. They found related terms were situated in close proximity - terms like Golden Gate Bridge, were close to Alcatraz Island, Ghirardelli Square, the Golden State Warriors, California Governor Gavin Newsom, and others.
Until there is full transparency into how these models operate, it falls to us to manage and mitigate their risks - especially in a healthcare context. "While machines can draw attention to trending technologies, customer pain points, or particularly novel technologies, the final decision on how to proceed remains the responsibility of human innovation experts."
Take the time to craft an AI Risk policy for your organization - it can guide you on the best ways to incorporate AI into your products and operations.
These are still stochastic systems with a degree of randomness of the answer, and that is why we keep humans in the loop - augmenting human cognition rather than replacing it outright.
A Call to Action
You may have heard that AI is the “worst it will ever be.” While that remains to be seen, there is still no better time to start working with AI than now. Look at your workflows, and where you can augment your team with these generative tools.
Embrace change and design your process to avoid lock-in as LLMs continue to leap-frog each other in capability. Be flexible so that you can adopt more powerful or more affordable models as they become available.
Leverage the temperature setting to manage the creativity of the LLM’s output space. Dial it up in low-risk ideation sessions, but lower it when working on more high-stakes or patient-facing concepts.
Start small with a low-stakes challenge and augment your team using these techniques. You’ll be surprised at how fast you can move. Buckle up!
Applying Generative AI with the Double Diamond
The examples below offer sample use cases and prompts for applying Generative AI to the Double Diamond process.