Last week, we had the privilege of co-hosting with Digital Health Insider (DHI), an insightful roundtable discussion to learn all about "Innovating with AI in Healthcare Systems". Here, we delve into AI as a solution, when and how to use it in healthcare systems, the biggest challenges in these organizations, how to overcome them with AI, and different approaches to healthcare systems.
The roundtable was moderated by Dael Stewart, Managing Director for North America at Light-it, and our panel of industry experts included Rachel Tornheim, Joe Ferrara, and Scott Nelson.
- Rachel Tornheim is an experienced professional in the healthcare sector, having worked in health tech startups, health systems, and payer organizations in New York City. Most recently, she led the consumer digital innovations team at Mount Sinai Health System.
- Joe Ferrara has extensive experience in sales and customer service within the healthcare industry. He has held senior positions at innovative life sciences startups and currently leads customer service at PharmASSIST Healthcare Dashboards (PHD).
- Scott Nelson is an Associate Professor in Biomedical Informatics at Vanderbilt University Medical Center. He specializes in medication safety informatics and also directs Vanderbilt's Master of Science in Applied Clinical Informatics program.
The Rise of AI in Healthcare: what's been happening recently?
Innovation as a concept or practice isn’t new in healthcare, but the pace and visibility of AI-driven advancements have dramatically increased. AI's role has shifted from a behind-the-scenes function to a central, transformative force in healthcare delivery and management. It’s no longer about creating complex new healthcare approaches that take years but more about accelerating, streamlining, and making healthcare more accessible.
But what exactly has been happening? For example, Dael shared that:
- At least 110 Health Systems have set up innovation centers in the US; this is enabling the acceleration of new products and services, often from providers and 'intrapreneurs' within those Health Systems.
- AI is powering automated screening of CT scans to provide earlier detection of lung cancer.
- x12 reduction in time to treatment was achieved by the Mayo Clinic Center for Innovation by applying AI tools and algorithms
- 1B was the IPO for a spin-off from Penn Medicine Innovation Center
So, why is the rapid growth of AI so important to healthcare users across-the-board?
Here, Scott emphasized that AI's proliferation is driven by several critical factors:
- Healthcare Workforce Shortages: There are significant shortages across various healthcare roles, including nurses, physicians, and pharmacists. AI can help alleviate some of these pressures by improving efficiency.
- Clinician Burnout: High burnout rates among healthcare professionals necessitate solutions that can reduce workload and streamline processes. According to a survey by the American Medical Association, about 63% of physicians have experienced burnout in the past year.
- Cost Management: With healthcare accounting for 18% of US GDP, there is intense pressure on Health Systems to be more efficient and reduce costs. AI offers potential savings through increased efficiency and better resource utilization. Joe expanded on this topic and explained that there is a great deal of value in AI solutions that focus on handling back-end and administrative tasks that would otherwise be done by doctors, nurses, and other clinical staff. These tools allow clinicians to spend less time in front of screens and more time in front of patients. “In my view, this is the low-hanging fruit that can demonstrate the value of AI and show immediate ROI”, he said.
- Complexity of Healthcare: The exponential growth in medical knowledge, which is estimated to double every 73 days, requires advanced tools to manage and utilize this information effectively. AI can assist in synthesizing vast amounts of data to support clinical decisions.
So, AI has this promise of helping to improve efficiency, decrease costs, and manage these complexities so that clinicians can take the best possible care of our patients.
Who are the key players in health systems, and what are their innovations?
Some of the nation’s largest health systems have been early adopters of AI, and Joe shared a few examples of how they are leading the way:
- Banner Health: Based in Phoenix, Arizona - Banner Health has partnered with Regard to streamline electronic health record (EHR) access, allowing physicians to spend more time with patients.
- Johns Hopkins: Collaborating with GE Healthcare, Johns Hopkins uses predictive AI in emergency rooms to expedite patient triage and admission, significantly improving efficiency. Since implementation, the facility has assigned patients admitted to the ER to beds 38% faster!
- Northwell Health: This New York-based health system has implemented AI solutions from Aidoc across its 17 hospitals to optimize clinical workflows and improve patient care.
These examples illustrate how AI is not just a theoretical concept but a practical tool delivering real-world benefits in healthcare.
How can we address AI Legal and Ethical Challenges in Healthcare
Knowing the healthcare industry's risk-averse nature, we emphasized the importance of addressing legal and ethical challenges as AI becomes more prevalent. We are creating new areas that haven't been considered or tested before, so how do privacy, security, general legal protections, and considerations play into this sea-change?
“Hospitals moving too fast is not a concern that I have”, Rachel shared. Health systems tend to be slow-moving due to the size of the organizations, and they also tend to be quite risk-averse due to operating in a highly regulated industry where auditors often show up unannounced. Thus, Health systems typically have a lot of controls to make sure there is sign-off from teams that oversee privacy, security, and other considerations when implementing new technology, and AI is no exception. She explained that “a lot of health systems are putting together specific committees to evaluate and oversee the introduction of new AI technologies, and I’m confident that representatives from the privacy/security and legal departments are represented”.
How and When to use AI in healthcare systems
Moreover, Rachel Tornheim shared a comprehensive framework for assessing the risk associated to implementing AI in healthcare systems. This framework shows various drivers of risk, spanning from less risky to more risky implementations.
Firstly, Rachel discusses the task type AI is intended to support. At the lower end of the risk spectrum are back-office tasks, such as billing or procurement, which have minimal impact on patients. Moving along the continuum, front-office tasks, like scheduling, entail patient interaction, thus warranting more concern over potential errors. Further up the risk scale are patient care operations, which involve administrative tasks related to care delivery but are not directly clinical. Finally, the highest-risk category encompasses the actual delivery of care, including clinical decision-support tools that make care recommendations or analyze medical images.
The second driver of risk pertains to the type of AI model being implemented. She distinguishes between deterministic and non-deterministic models. Deterministic models yield consistent outputs for a given input, as the rules are explicitly coded. Conversely, non-deterministic models, such as machine learning algorithms, generate uns predetermined outputs, leading to higher variability and risk.
The level of oversight constitutes the third driver of risk. Low-risk implementations entail full oversight, with every AI recommendation reviewed by a human. Intermediate options may involve partial oversight, wherein certain model outputs are reviewed based on predefined criteria. Conversely, high-risk scenarios may involve no oversight, although such implementations are rare.
Rachel explained that real-world implementations of AI in healthcare typically involve a mix of risk levels across these elements. For instance, high-risk tasks may necessitate greater oversight, while lower-risk tasks may be associated with less oversight. This nuanced approach allows healthcare systems to tailor their AI implementations according to the specific risks involved, balancing innovation with patient safety and regulatory compliance.
Highest value use-cases for AI in healthcare today
We've already discussed how AI can reduce administrative burdens by operating behind the scenes. Building on that, Joe explained that there are significant opportunities in diagnostic tools, though they come with a need for caution.
A compelling example is Rad AI, a company using AI to generate radiological impressions tailored to each radiologist's unique language style. The AI learns individual preferences and generates reports, saving substantial administrative time. Rad AI claims this could save up to 60 minutes per shift per radiologist, significantly boosting efficiency and accuracy.
These advancements are not just about efficiency but also about improving the quality of patient care. A critical aspect of successful AI implementations is the oversight component, ensuring AI can augment rather than replace clinical expertise. This collaboration between AI and clinicians helps ease workloads, potentially reduce burnout, and enhance patient outcomes.
Another set of intriguing use cases comes from Light-it's Innovation Lab: Puppeteer and CompliantChatGPT. Puppeteer empowers healthcare organizations to use conversational AI to enhance the experiences of both providers and patients. It significantly improves efficiency by integrating seamlessly into existing workflows and meeting patients and providers where they are.
CompliantChatGPT is another innovative platform enabling the use of OpenAI GPT models in a HIPAA-compliant manner. This ensures that AI can be leveraged securely within the healthcare sector.
Light-it is actively working on bringing these and other products into healthcare systems to drive further innovation and improve patient care.
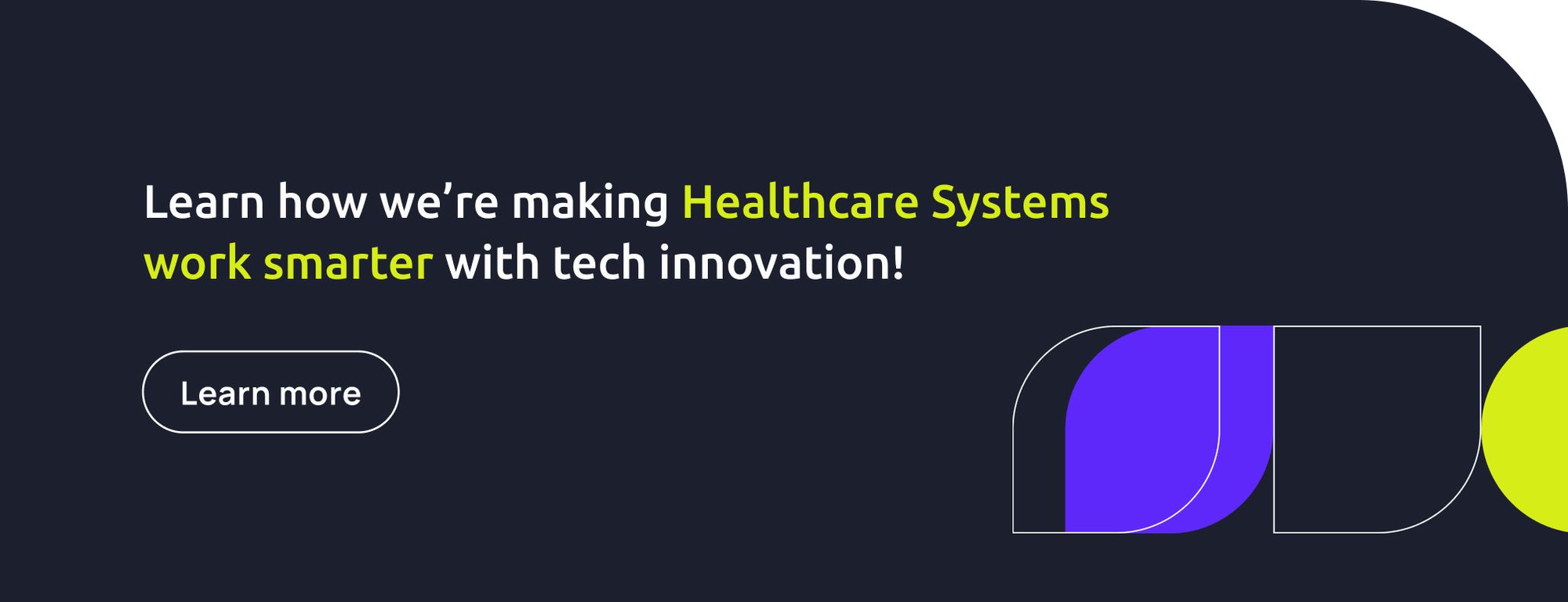
Best Practices for Harnessing Emerging AI Technologies in Healthcare
When considering AI models and algorithms, Scott explained that we can approach it similarly to how a pharmacist considers new medications entering the market. For a new drug to reach the market, safety, and efficacy must be established, and the FDA has set up a framework for software as a medical device that follows a similar review structure to that of medical devices or drugs. This framework is crucial for ensuring that AI applications in healthcare are safe and effective for patients. We’ve seen cases where AI models were implemented and, unfortunately, led to increased mortality rates, necessitating their shutdown. Therefore, focusing on lower-risk administrative tasks for AI can yield substantial benefits without compromising patient safety.
So, to effectively leverage AI while ensuring patient safety and maintaining operational efficiency, he highlighted several best practices to follow
Dos:
- Thorough Evaluation: Take the time to thoroughly evaluate any AI model or product, especially concerning cybersecurity and patient safety. As Rachel mentioned, these aspects are paramount.
- Cost and Maintenance Awareness: Be mindful of the ongoing costs and maintenance requirements, as AI models tend to drift over time. Plan for long-term upkeep and updates.
- AI Governance: Establish an AI governance group or structure to review and approve AI tools. This ensures a systematic and safe approach to AI implementation.
- Critical Thinking and Skepticism: Apply critical thinking and maintain a healthy dose of skepticism towards AI claims. Not all AI applications are necessary or beneficial.
Don'ts:
- Avoid the Hype: Don't get swept up in the AI hype. Just because a product can incorporate AI doesn't mean it should. Many tasks, like using a toothbrush or children's toys, don't require AI.
- Overly Complex Tasks: Don't focus on using AI for overly complicated tasks or on completely replacing clinicians. AI should augment, not replace, human expertise. A better term for AI is augmented intelligence, which emphasizes its role in facilitating workflows rather than replacing professionals.
Radiology is a prime example. Initially, there was a push to replace radiologists with AI, but it became clear that while AI can excel at specific tasks, it can't perform the full range of radiological functions. Instead, the focus has shifted to how AI can assist radiologists in their work, making their tasks easier and more efficient.
How Innovation Centers are Driving Growth in Healthcare Systems through AI
Moving forward in the roundtable, Rachel explained all about innovation centers in health systems, and started off by differentiating the two main types of innovation centers within health systems:
- One type focuses on building solutions internally, sometimes with the aim of spinning them off into separate companies. For these centers focused on building solutions, the track record is mixed. Some health systems have successfully created and spun out new AI products. Others have attempted this but ultimately pulled back, realizing that their core competency might not lie in building and launching software products.
- The other type acts more like internal consultants, researching external solutions and advising internal departments on their implementation. This second type of innovation center, which functions as a dedicated team assessing and implementing AI technologies, can be very effective. These groups typically survey the landscape, research various solutions, and assess their applicability to their particular institution. They work closely with different departments to understand their pain points and needs, identify suitable solutions, manage the assessment process, and oversee the implementation and measurement of success.
This approach is beneficial because, without a dedicated team, the responsibility falls to the leadership of individual departments, such as the call center or radiology department heads, who may struggle to find the time or may lack the necessary skills. Having a dedicated team ensures a more strategic and focused effort in implementing new solutions.
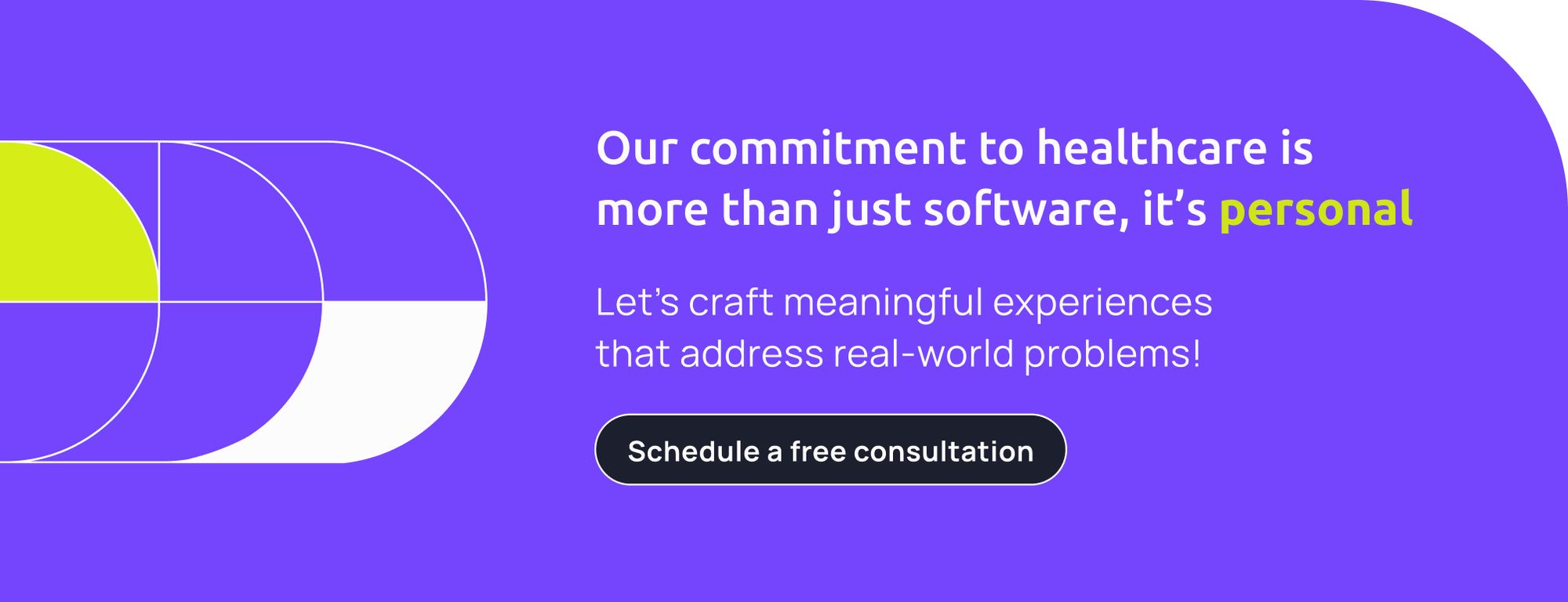
The biggest challenge in Healthcare Systems & How to overcome them with AI
We've discussed in the roundtable when and how to use AI in healthcare systems, emphasizing that some of the most immediate impacts might be less about diagnostics and more about supporting frontline workers and providers. Here, one critical area is physician burnout, and according to a recent survey by Athena Health, over 90% of physicians report feeling burned out regularly, primarily due to the paperwork they must handle.
Additionally, Dr. Jesse Ahrenfeld, President of the AMA, projects a shortage of 100,000 physicians in the US by 2034. Staff shortages are a significant issue here. According to HLTH Conf, by 2034, we could face a shortage of up to 124,000 physicians. The situation is even more dire for nurses, with an estimated 600,000 expected to leave the profession by 2027. Therefore, Rachel explained this trend and shared her observations and insights on how AI might help reverse it.
“Clinician shortages are a well-documented challenge, but fortunately, several AI tools are emerging to help address this issue” she elaborated:
- For physicians, AI tools can summarize patient charts, speeding up their preparation for patient visits. Additionally, ambient AI tools can listen during patient visits and draft the notes for physicians. Creating these notes is a major contributor to "pajama time," where doctors catch up on administrative work at home, leading to burnout and job dissatisfaction.
- For nurses, AI tools can draft responses to patient questions using generative AI, especially when they use text-based applications to communicate. This saves nurses time as they can edit and send pre-drafted messages instead of writing each one from scratch. Machine vision tools that analyze radiology or pathology images also improve efficiency in those departments by allowing clinicians to review and edit AI-generated drafts rather than starting from scratch.
These AI tools aim to address clinician shortages in two main ways:
- By increasing efficiency, they enable healthcare providers to do more with fewer people.
- By reducing the administrative burden on clinicians, they enhance job satisfaction and potentially reduce turnover, which is a significant factor in clinician shortages.
Another interesting AI application is in shift management for departments like nursing and phlebotomy. A company recently showcased an AI system that dynamically adjusts incentive pricing for open shifts, offering these shifts to available staff more efficiently. This approach has been shown to reduce reliance on external agencies and increase internal staff satisfaction by offering more overtime opportunities, thus helping to alleviate staff shortages.
Moreover, Scott added the significant impact of voice-to-text technology in healthcare and anticipated further advancements with large language models (LLMs) and generative AI. However, amidst the excitement surrounding technological innovations, it's crucial to address the challenges of implementation.
One notable example shared in the discussion was the use of an AI algorithm to interpret chest X-rays, aimed at reducing the workload for radiologists. Yet, this initiative inadvertently led to an increase in complexity, as radiologists were presented with more intricate images, resulting in heightened dissatisfaction within the profession.
Conversely, the application of generative AI in composing draft messages for patient communications showcased promising outcomes. Although it didn't necessarily reduce the time spent on messages, it significantly alleviated cognitive burden, offering a more streamlined and satisfying experience for healthcare professionals. Conversely, the application of generative AI in composing draft messages for patient communications showcased promising outcomes.
These generative messages reduce some of the friction experienced by healthcare professionals, providing a starting point and prioritizing responses. Scott elaborated on this aspect, highlighting how these tools allow users to quickly navigate through responses, enabling them to refine and build upon initial drafts. Even if the time taken to reply to messages remains the same, there is a notable decrease in cognitive burden, offering a more efficient and satisfying experience.
So, as advancements continue, it becomes imperative to prioritize not only efficiency but also the overall user experiences and psychology of how we're implementing the AI, ultimately driving positive outcomes in patient care.
Complexities of Selling into Healthcare Systems
Up until this moment in the roundtable, our panel of experts spoke on how health systems are applying AI and what their needs and wants are. Yet, having these organizations implement novel and external solutions, products, and ideas is not easy.
They're large institutions, oftentimes entrenched bureaucratic risk averse, so processes with them tend to be long and specific. However, the impact these systems have because of their size and scale, and the value they bring, is immeasurable. Therefore, Rachel, who has previously worked in a health system, explained how these systems are typically set up, and what their motivations are.
Here, it’s important to bear in mind that healthcare systems tend to be very large organizations. Even a single-site healthcare system can have around 10,000 employees, such as Sinai or NYU with 40 to 50,000. Larger ones, like Northwell, can have up to 80 or 100,000 employees. This organizational magnitude has significant implications.
- Firstly, employees in different departments may not know each other, and they are more likely to have very different goals compared to smaller organizations, where there is often a greater sense of everyone working towards the same goal.
- A second characteristic of healthcare systems is their age. Many of them have been around for over a century, meaning they have established processes that have been in place for a long time. This can make it more effective to work within their existing processes rather than trying to force changes due to their more traditional culture.
- Additionally, healthcare systems tend to be financially constrained, with low or even non-existent profit margins. This means they do not have funds readily available, so any investment in an external solution must be backed by a solid financial case demonstrating how it will benefit their budget in the long term. Thus, the importance of having a clear and quick return on investment (ROI) to capture the attention of the financial leaders of these systems. Without a clear perspective of how a solution can generate savings or additional revenue within a relatively short period of time, interest is likely to wane. Rachel explained, “improving quality, improving patient experience, it’s great, but if you cannot accompany that with cost savings or additional revenue, or translate that into a financial ROI. It's likely to be a nonstarter”.
- Last but not least, healthcare systems are highly regulated. In addition to the usual healthcare industry regulations, these systems face frequent and unexpected audits, contributing to a cautious organizational culture.
“So all of these factors really contribute to health systems being slow-moving and risk-averse”, Rachel explained. That means it's harder to get in. But it also means that once you're in, you're in.
Strategic Approaches to Engaging Healthcare Systems
Our panelist, Joe Ferrera, has great experience selling to health systems in the past, so the end of the roundtable focused on strategies and recommendations to effectively approach these organizations:
- Openness to Conversations at All Levels: Joe emphasizes the importance of being open to engaging with individuals at all levels of a health system, particularly for early-stage companies. With healthcare systems being large and multifaceted entities, pinpointing the right contacts can be challenging. Early conversations allow for the discovery of pain points, which are crucial in assessing the fit of solutions. These dialogues not only reveal potential problems, but also help perfect pitches by addressing customer objections early on.
- Identification of Champions: As you navigate through the healthcare system, identifying champions within the organization becomes paramount. These champions are individuals who will be directly using your product or service regularly. Their endorsement can significantly influence decision-making processes, from initial discussions to contract negotiation and implementation. Investing time in nurturing these relationships can lead to long-term success.
- Ease of Collaboration: Joe underscores the importance of simplicity and ease of collaboration. Complexities inherent in AI solutions should not be compounded by cumbersome processes. Keeping presentations concise and focused, with 7 to 10 slides at most, allows for clarity of communication. By highlighting one or two key features or ideas, you can effectively convey the value proposition of your offering. Moreover, adapting to the contracting processes preferred by the health system demonstrates flexibility and fosters smoother collaboration.
- Pilot Projects and Low-Risk Initiatives: Pilot projects serve as effective entry points for startups aiming to demonstrate the value of their solutions. Joe outlines two successful approaches: the Freemium model and discounted introductory offers. These initiatives mitigate the perceived risk for healthcare systems, providing them with an opportunity to trial products with minimal financial commitment. Such initiatives not only facilitate revenue generation for startups but also help build trust and credibility over time.
- Strategic Outreach: In today's digital landscape, strategic outreach is key. Joe recommends leveraging platforms like LinkedIn for initiating conversations and relationship-building. LinkedIn offers a more conducive professional networking and knowledge-sharing environment unlike traditional email channels. By engaging prospects through personalized messaging and offering value-driven interactions, you can increase the likelihood of securing meetings and progressing discussions.
Joe's insights underscore the importance of adaptability, relationship-building, and simplicity in engaging with healthcare systems. By adopting these strategic approaches, startups can navigate the complexities of the healthcare landscape more effectively, ultimately leading to successful partnerships and collaborations.
Here, it's not just about the product but knowing your audience. How does this work for them to satisfy their business needs?
Evaluating AI Vendors for Healthcare Systems
Moving forward in the discussion, Scott Nelson provided valuable insights into the process of evaluating vendors, particularly in the context of healthcare systems. Here's a breakdown of his recommendations and strategies:
- Data-Driven Evaluation: Scott emphasizes the importance of data in the evaluation process. Vendors must demonstrate the ability to quantify the problem they address and provide evidence of their solution's efficacy. Establishing a clear ROI upfront is essential, along with understanding the organization's current state to determine if the proposed solution aligns with existing needs.
- Transparency and Information: Drawing an analogy with medication, Scott advocates for a model brief—a comprehensive document that provides essential information about AI models. This includes details on the data used for training, intended uses, potential side effects, fairness metrics, and maintenance requirements. Just as prescribers need detailed medication information, healthcare systems require transparency about AI models to make informed decisions.
- Cybersecurity and Privacy: Scott highlights cybersecurity as a top priority in vendor evaluation, especially in light of recent cybersecurity incidents in the healthcare sector. Vendors must demonstrate rock-solid cybersecurity measures and privacy protocols to safeguard sensitive patient data. Any implementation of new technologies or integrations must adhere to stringent cybersecurity standards to protect healthcare organizations from potential breaches. It's important to remember that you could have the best solution that has the ability to make such a fundamental impact, but if you can't do that in a way that protects privacy or that is secure, then there's no one out there that's gonna buy them.
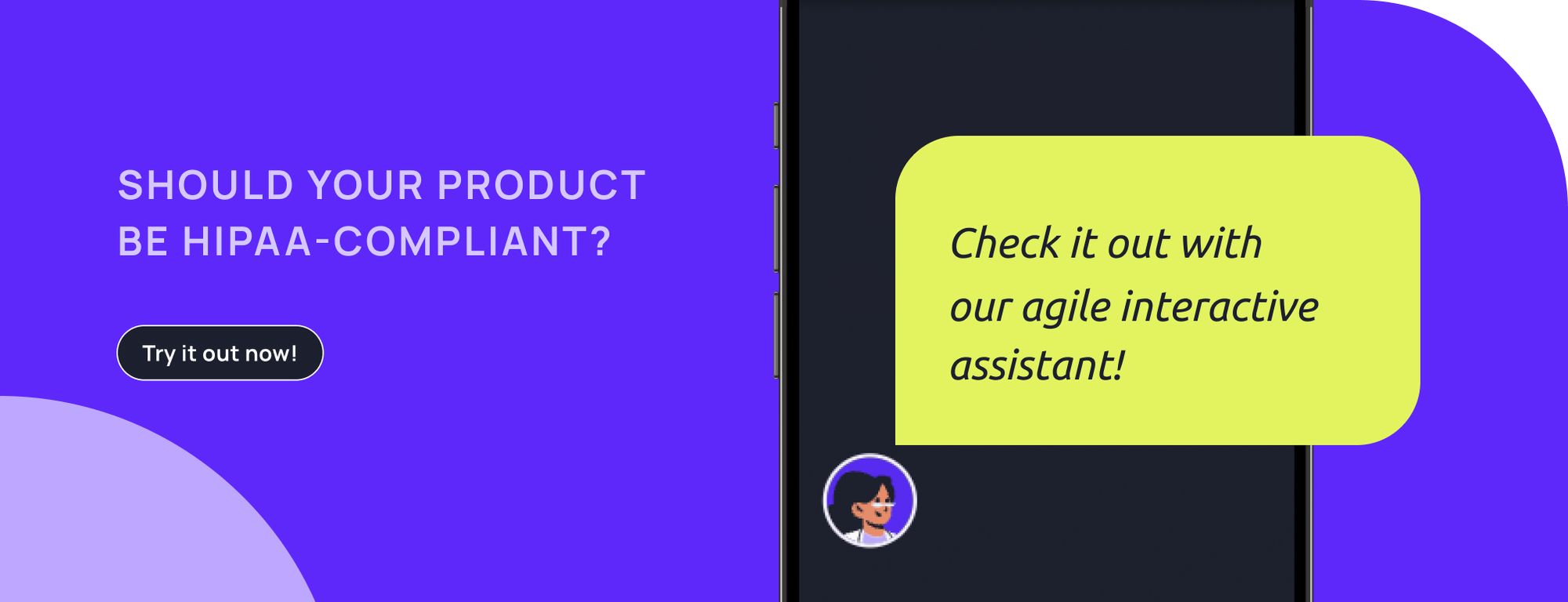
Differentiating Approaches in a Competitive Healthcare Landscape
How can you set yourself apart in an increasingly competitive landscape within the healthcare industry? Rachel shared strategies entrepreneurs and innovators can use to differentiate themselves and establish meaningful partnerships with health systems.
- Customized Pitching: Rachel emphasizes the importance of tailoring your pitch to each individual health system. Instead of presenting a generic solution, gather specific evidence from the target institution and incorporate it into your presentation. By addressing how your solution tackles issues unique to their organization, you demonstrate a deeper understanding of their needs and differentiate yourself from competitors.
- Personalized Financial ROI: When discussing financial return on investment (ROI), Rachel suggests making assumptions that are specific to the health system in question. This approach ensures that your ROI projections are realistic and resonate with the decision-makers. By aligning your financial metrics with the institution's circumstances, you enhance the credibility of your proposal.
- Relevant Case Studies: Selecting case studies that closely resemble the target health system's profile is crucial. You provide tangible evidence of your solution's effectiveness by showcasing success stories that mirror their challenges and objectives. This tailored approach resonates more strongly with potential partners, as they can envision the potential impact on their own organization.
- Mitigating Risk Through Customization: Addressing concerns about risk is essential in building trust with health systems. Rachel suggests customizing your risk mitigation strategy based on the institution's specific circumstances. By referencing past incidents or vulnerabilities and proposing tailored solutions to mitigate them, you demonstrate thorough research and a proactive partnership approach.
Q&A: AI Adoption in Healthcare: Urban-Rural Disparities and Equitability Concerns
To finish the roundtable, we passed on to the Q&A section, where we mainly focused on the following question that an attendee sent us: With the U.S. population split evenly between urban and rural areas, how will AI impact health disparities in these regions? Are rural healthcare systems slower in adopting AI tools?
Rachel tackled this urban-rural AI impact and equitability concern by noting a crucial difference between urban and rural health systems. She pointed out that urban areas see more competition among health systems due to the presence of multiple providers, leading to a greater motivation to adopt new technologies to address staffing shortages and burnout. On the other hand, rural health systems, lacking such competition, may wait for others to lead in adopting new technologies rather than being at the forefront themselves.
Finally, Scott Nelson concluded by expressing his curiosity about how things will unfold over time. He mentioned the existing health disparities between rural and urban areas and pointed out that as more rural centers close, telehealth could become a way to bridge the gap. However, he also questioned how AI adoption might affect things, such as efficiency gains and cost reductions. Scott wondered if AI could create a divide between healthcare organizations, and he emphasized the need to keep an eye on how these changes play out in society.
Subscribe to DHI and stay tuned for the next discussion:
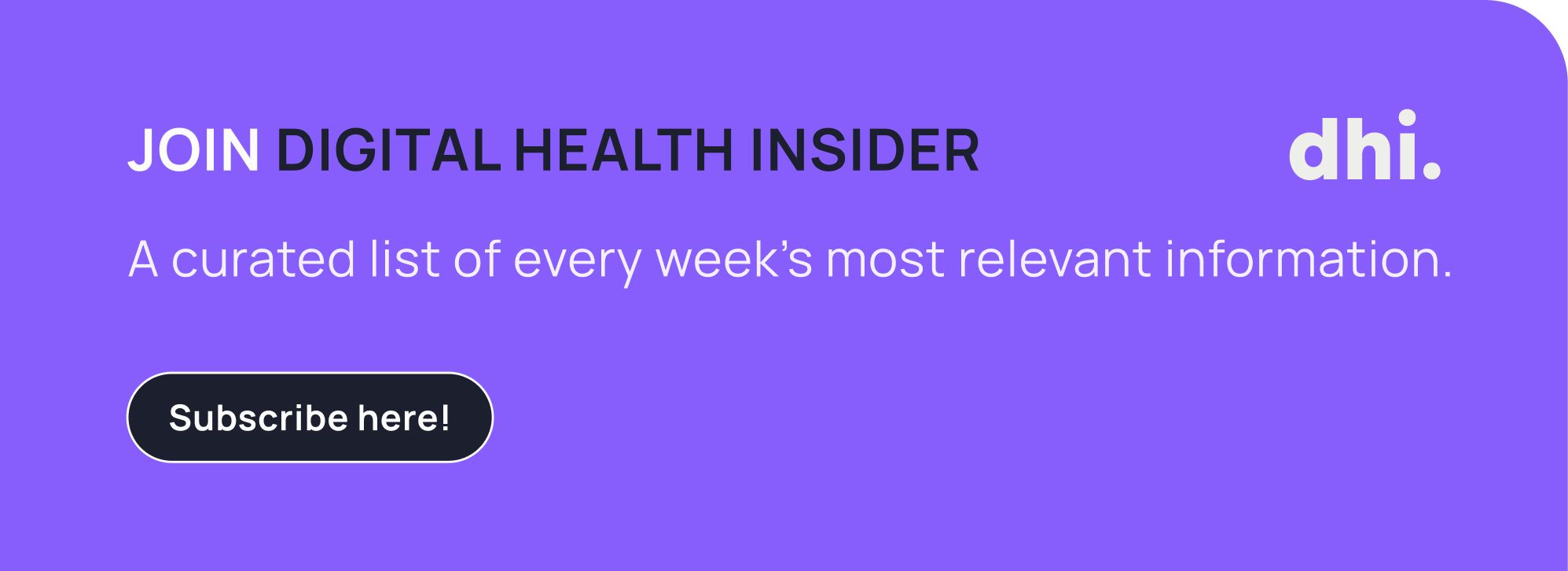
It was such an exciting opportunity to share with top industry experts from various points of view! Huge thanks to the +200 attendees who joined us virtually. Thank you as well, Rachel Tornheim, Joe Ferrara, Scott Nelson, and Dael Stewart, for sharing such interesting insights and joining DHI and Light-it to shed light on critical topics. Stay tuned for the next DHI and Light-it roundtable!
For more articles on AI in healthcare, check out Light-it’s blog posts!