The responsible regulation of artificial intelligence (AI) has become a critical factor in the healthcare scene.
AI governance refers to the set of policies, procedures, and guidelines designed to ensure the responsible and ethical use of AI systems within an organization. It encompasses the principles, frameworks, and mechanisms that govern the development, deployment, and management of AI applications, focusing on mitigating risks, ensuring compliance with regulations, and promoting transparency and accountability.
Within the healthcare ecosystem, AI governance plays an important role in safeguarding patient privacy, optimizing clinical decision-making, and enhancing the quality and safety of patient care. As healthcare organizations increasingly rely on AI-driven technologies to streamline processes, improve diagnostic accuracy, and personalize treatment plans, the need for robust governance frameworks becomes imperative. An AI governance framework provides a structured approach to managing AI-related risks and challenges, guiding organizations in the ethical and effective implementation of AI solutions.
This article explores the connection between AI governance and healthcare, focusing on best practices for ensuring data privacy and algorithm transparency in AI-driven healthcare applications. We delve into key strategies for mitigating bias, obtaining informed patient consent, and cultivating trust in AI technologies. By providing actionable guidance and recommendations, we aim to empower healthcare organizations to navigate the challenges of AI governance successfully and harness the transformative potential of AI in healthcare.
1. Data Privacy in Healthcare AI
The advent of electronic health records (EHRs) and digital health platforms has led to an unprecedented surge in the volume and diversity of healthcare data. While this data explosion offers immense potential for advancing patient care through AI-driven innovations such as personalized treatment plans, predictive analytics, and early disease detection, it also introduces significant challenges concerning data security and patient privacy.
Patient health data is highly sensitive and must be handled with the utmost care to ensure compliance with regulations such as the Health Insurance Portability and Accountability Act (HIPAA) in the United States and similar laws worldwide. HIPAA establishes strict guidelines for the collection, storage, and transmission of protected health information (PHI), mandating security measures to safeguard against unauthorized access and disclosure. Compliance with such regulations is non-negotiable for healthcare organizations, underscoring the critical importance of stringent data privacy protocols to uphold patient confidentiality and foster trust.
However, regulatory adherence alone is insufficient. Healthcare AI developers and organizations must proactively embrace a comprehensive suite of best practices to fortify data privacy defenses. Key elements of these best practices include:
- Cutting-Edge Encryption Techniques: Implementing advanced encryption methods is key to ensuring the security of healthcare data, both at rest and in transit. By encrypting data using robust algorithms and cryptographic protocols, organizations can safeguard sensitive information from unauthorized access or interception.
- Stringent Access Controls: Restricting data access to authorized personnel is critical in preventing unauthorized disclosure or misuse of patient information. Implementing stringent access controls, such as role-based access mechanisms and multi-factor authentication, helps ensure that sensitive data is accessible only to individuals with legitimate reasons and permissions.
- Regular Audits and Monitoring: Continuous monitoring and auditing of data access and usage are essential components of effective data privacy governance. By conducting regular audits, organizations can identify potential security gaps or compliance issues, allowing for prompt remediation and strengthening of data protection measures.
- Utilization of Compliance Assessment Tools: Light-it has developed an innovative solution known as the HIPAA Checker, designed to streamline the compliance assessment process for healthcare AI developers and organizations. This interactive chat-based tool serves as a comprehensive guide, assisting users in determining whether their digital health products, startups, or ideas require HIPAA compliance. By leveraging the HIPAA Checker, organizations can navigate the intricate landscape of regulatory requirements more effectively, identifying areas of non-compliance and implementing remedial measures promptly.
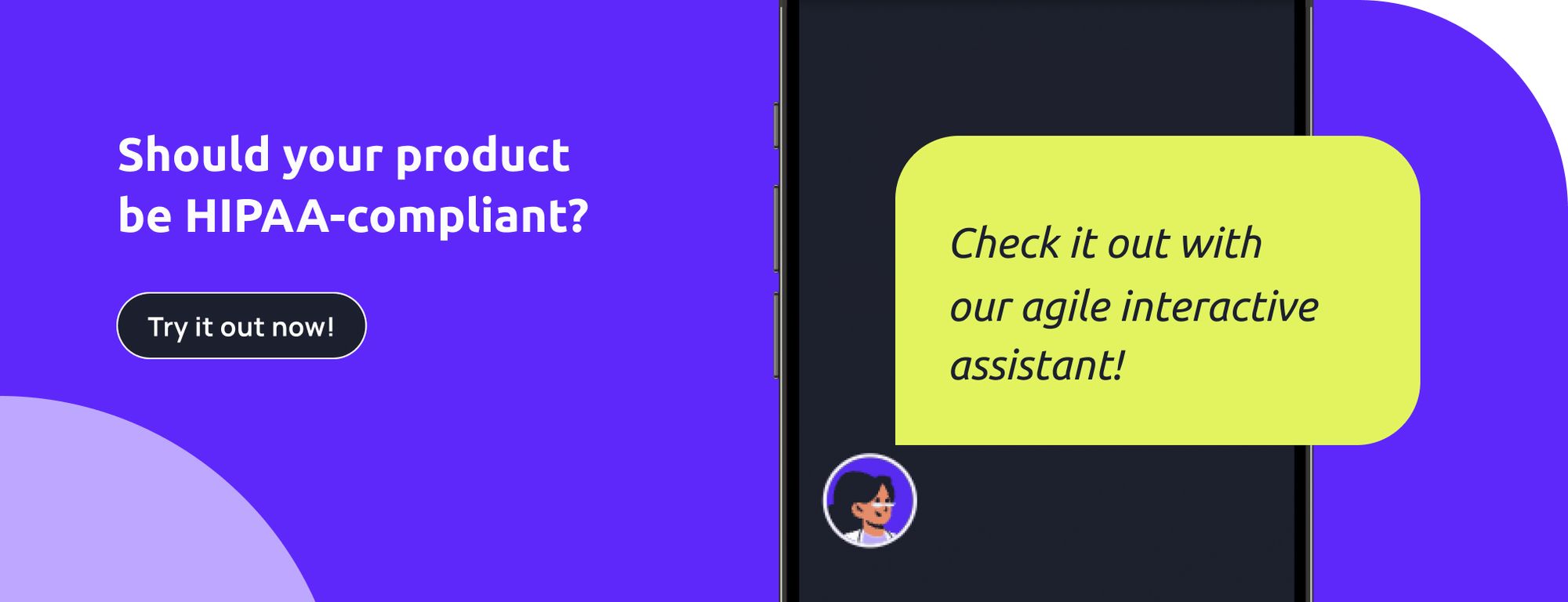
By adopting these proactive measures and integrating them into their data privacy frameworks, healthcare AI developers and organizations can reinforce the integrity and confidentiality of patient information, thereby bolstering trust and confidence in AI healthcare solutions.
2. Strategies for Ensuring Algorithm Transparency
Algorithmic transparency is essential for building trust in AI-driven healthcare applications. Transparency allows stakeholders, including patients, healthcare providers, and regulatory bodies, to comprehend the functioning of algorithms, the data they utilize, and the factors influencing their decision-making processes. Transparent algorithms foster accountability and enable stakeholders to assess the fairness, accuracy, and reliability of AI-driven recommendations and predictions.
In order to achieve algorithm transparency, healthcare organizations must adopt key strategies throughout the lifecycle of AI systems:
- Clear Documentation: Comprehensively documenting algorithms and model architectures ensures transparency and enables stakeholders to understand how AI systems operate.
- Disclosure of Training Data: Transparently disclosing the sources of training data and preprocessing techniques enhances trust and accountability in AI in healthcare.
- Validation against Benchmarks: Validating algorithm performance against established benchmarks and regulatory requirements assures stakeholders of the reliability and effectiveness of AI systems.
- Utilization of Visualization Tools: AI-driven healthcare solutions can be better understood and trusted by non-technical stakeholders when complicated algorithmic concepts are communicated with the use of visualization tools. By converting data and algorithms into accessible formats such as charts, flowcharts, heatmaps, tree diagrams, and interactive dashboards, stakeholders can gain a clearer understanding of how AI systems function, empowering them to assess the fairness and reliability of these systems.
Moreover, transparency in algorithmic decision-making is vital for ensuring equitable healthcare outcomes and addressing concerns about bias and discrimination. The Coalition for Health AI (CHAI™), plays a significant role in advancing transparency and accountability in AI governance for healthcare. By collaborating with industry experts and stakeholders, CHAI™ develops frameworks and guidelines to promote the adoption of transparent AI systems in healthcare, fostering trust and confidence among patients, providers, and policymakers.
3. Mitigating Bias in Healthcare AI
Algorithmic bias, within the context of AI, refers to the unjustified and systematic favoritism exhibited by AI systems towards particular outcomes or groups. This bias is a serious problem for the healthcare sector since it can result in mistakes or discrepancies in patient care, which could further reinforce already-existing disparities in healthcare. Bias can originate from a variety of sources, including biased training data, algorithmic design choices, and systemic prejudices embedded in healthcare organizations. A comprehensive strategy, including data collection, algorithm design, and continual monitoring and evaluation, is needed to address bias.
To mitigate bias in AI-driven healthcare applications, healthcare organizations must implement a range of strategies:
- Rigorous Data Preprocessing: Thorough preprocessing of training data is essential to identify and mitigate biases effectively. This involves scrutinizing datasets for inherent biases and employing techniques to mitigate their impact on model training.
- Algorithmic Fairness Assessments: Conducting fairness assessments on algorithms helps identify and rectify bias in model predictions. By evaluating the fairness of algorithmic outcomes across different demographic groups, organizations can ensure equitable treatment for all patients.
- Ongoing Monitoring and Evaluation: Continuous monitoring and evaluation are crucial for detecting and addressing bias in real-world deployment. Healthcare organizations must establish mechanisms to monitor algorithmic performance and intervene promptly to rectify any instances of bias that may arise.
- Interdisciplinary Collaboration: Collaborative efforts involving data scientists, clinicians, ethicists, and domain experts are indispensable for designing and deploying AI systems that prioritize fairness, equity, and patient safety. By drawing on diverse perspectives and expertise, organizations can develop AI solutions that are sensitive to the needs and values of diverse patient populations.
- Promotion of Diversity and Inclusion: Advancing diversity and inclusion within AI development teams is paramount for mitigating bias and enhancing algorithmic fairness. By fostering a diverse and inclusive work environment, organizations can leverage a broader range of perspectives and lived experiences to inform the design and evaluation of AI algorithms, thereby promoting innovation and equity in healthcare delivery.
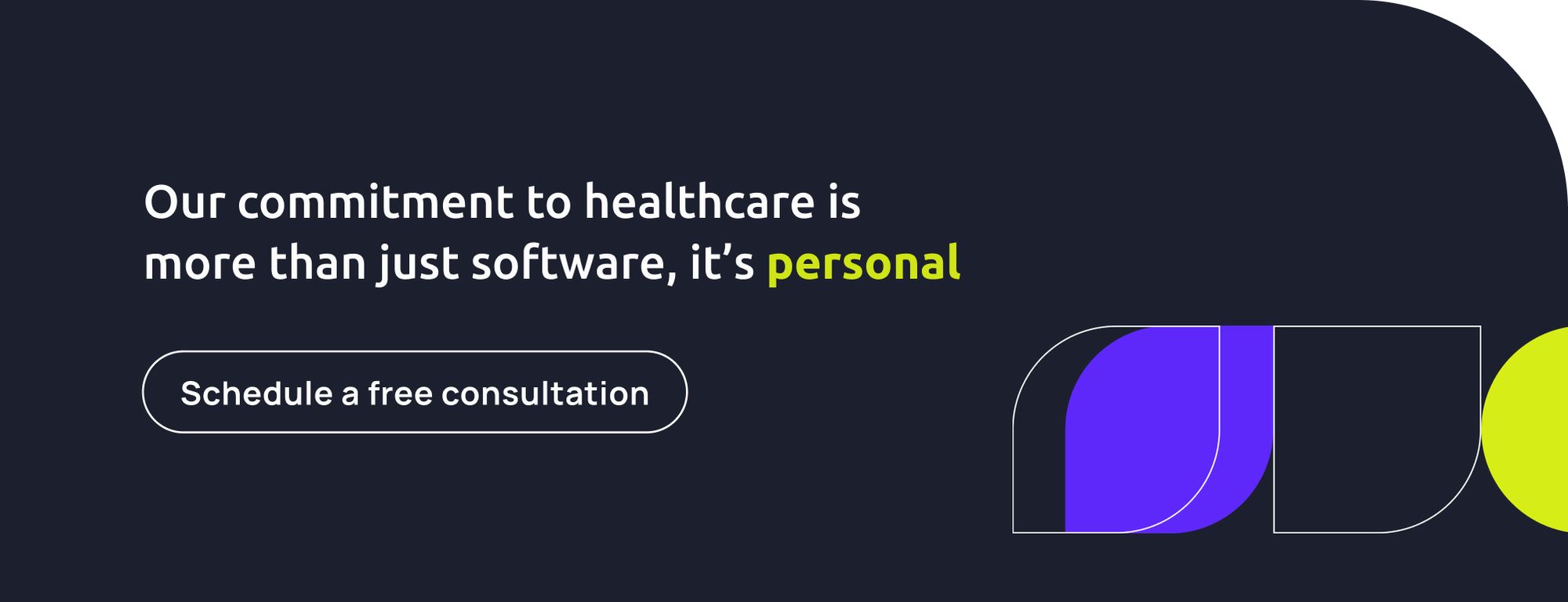
4. Ensuring Informed Patient Consent
Informed patient consent stands as a fundamental principle in the ethical deployment of AI in healthcare. Patients deserve transparency regarding how their data will be utilized in AI-driven healthcare applications and the opportunity to provide explicit consent for its usage.
Achieving informed consent requires clear and transparent communication regarding the purpose of data collection, the types of data gathered, its intended use, and the potential risks and benefits involved.
To uphold informed patient consent in AI-driven healthcare applications, healthcare organizations should adhere to best practices:
- Transparent Communication: Providing clear and accessible information about data collection and usage practices is critical. It must be ensured that patients understand how their data will be utilized in AI-driven initiatives and the implications of consenting to its use.
- Patient Empowerment: Offering patients control over their data is essential. Mechanisms such as consent preferences and data-sharing agreements enable patients to exercise autonomy over their health information, ensuring that their preferences and privacy concerns are respected.
- Ongoing Communication and Transparency: Maintaining open channels of communication throughout the patient-provider relationship is vital. Healthcare organizations should foster transparency by keeping patients informed about how their data is being utilized and any changes in data usage practices.
- Innovative Tools and Approaches: Leveraging innovative tools and approaches can enhance the consent process. Interactive consent forms and patient decision aids empower patients to make informed choices about their participation in AI-driven healthcare initiatives, ensuring that their preferences and concerns are effectively addressed.
By prioritizing transparent communication, patient empowerment, ongoing transparency, and the adoption of innovative tools, healthcare organizations can facilitate the informed consent process in AI-driven healthcare applications, thereby upholding patient autonomy and promoting ethical data usage practices.
Conclusion
The integration of artificial intelligence (AI) into healthcare is remodeling patient care and clinical decision-making. However, the responsible governance of AI in healthcare is critical to ensure ethical and effective implementation while safeguarding patient privacy and promoting transparency and accountability.
As we continue to advance AI technologies in healthcare, it is imperative that we remain vigilant in standing up for the principles of responsible AI governance. This includes the implementation of an AI governance framework, which provides a structured approach to managing AI-related risks and challenges, guiding organizations in the ethical and effective implementation of AI solutions.
By ensuring that the benefits of AI are realized while minimizing potential risks and maximizing societal benefit, we will be able to fully employ AI to transform the way healthcare is provided and improve the lives of individuals worldwide. Embracing a culture of transparency, accountability, and patient-centricity will be essential in achieving this goal.