As we increasingly apply Artificial Intelligence (AI) in the digital health sector, the value of data as the lifeblood for developing powerful, efficient, and effective AI solutions cannot be overstated. However, sourcing the right data is a challenge. In this post, we will explore five key strategies to source data for your digital health AI product.
To begin with, let’s go back to the basics and don’t take anything for granted.
What are data sources?
A data source is essentially where the information originates. In simpler terms, it's anything that provides the data you're using. There are many different types of data sources; here are a few examples:
- Databases: These are structured data collections that are electronically stored and organized.
- Spreadsheets: These are computer files that organize data in rows and columns.
- Text files: These are files that contain plain text.
- Sensor data: This is data collected from physical devices like thermometers or accelerometers.
- Web data: This is information that is publicly available on the web.
In digital health and AI-powered health products, data sources are the fuel that drives insights and innovation. They are the foundation for AI advancements in digital health. By using this data responsibly and ethically, AI is already revolutionizing healthcare by making it more proactive, personalized, and efficient.
Let’s explore 5 ways in which digital health companies can source data to bring their AI product to life: using public datasets, collaborating with medical care providers, harnessing IoT and mobile devices, generating synthetic data, and purchasing data from brokers.
Public Datasets
Public datasets serve as invaluable resources when embarking on data-driven projects in the healthcare sector. These treasure troves of information, such as the MIMIC-III critical care database and the Cancer Imaging Archive, offer vast quantities of data, often free of charge, presenting an ideal launchpad for healthcare research and development initiatives.
Yet, the utilization of these datasets is not without challenges. To comply with privacy regulations like the Health Insurance Portability and Accountability Act (HIPAA), the data is anonymized and stripped of certain specifics, thereby potentially limiting its applicability in certain research contexts. It is also crucial to remember that even when handling anonymized and de-identified data, we should uphold the highest ethical standards.
Let's dig into some key public datasets and resources that can guide the journey into healthcare data analysis:
- Medicare Public Use Files (PUFs): These resources offer insights into Medicare's patterns of utilization, payment structures, and prescription drug usage, among other areas.
- HealthData.gov: A government-backed website that grants access to a plethora of health data sets. These include information related to hospital comparisons, insurance, and a variety of health surveys.
- National Health and Nutrition Examination Survey (NHANES): This CDC-run initiative provides data on a broad spectrum of health and nutrition metrics in the US.
- Behavioral Risk Factor Surveillance System (BRFSS): Another CDC-led program, the BRFSS collects state-centric data on preventive health practices and risk behaviors associated with chronic diseases, injuries, and preventable infectious diseases.
- National Healthcare Quality and Disparities Reports (NHQDR): These reports offer a holistic picture of the healthcare quality received by the general U.S. population, highlighting disparities in care across different racial, ethnic, and socioeconomic groups.
- Tuva Health: Part of the Tuva Project, this set of data marts and terminology collections help transform healthcare data for analytic purposes. With marts focusing on Acute Inpatient, Chronic Conditions, Readmissions, and Service Categories, among others, Tuva Health supports dbt version 1.3.x or higher. It is compatible with data warehouses like Snowflake, Redshift, and BigQuery.
- Centers for Disease Control and Prevention (CDC): The CDC offers a variety of public health datasets through its multiple platforms:
- Data.CDC.gov: This repository houses all available data sets that come with a Socrata Open Data API. The categories range from Child and Flu Vaccinations to Health Statistics and Injury & Violence.
- Chronicdata.cdc.gov: This site provides data and indicators relating to chronic disease and health promotion.
- CDC.gov/data: Offers diverse data, statistics, and tools sorted by CDC topic area.
- National Center for Health Statistics: Provides downloadable public-use data files sourced from NCHS surveys and data collection systems.
- WISQARS™: An interactive database that provides fatal and nonfatal injury, violent death, and cost of injury data.
- National Notifiable Diseases Surveillance System: A nationwide collaboration for sharing notifiable disease-related health information.
- VaxView: A comprehensive resource for vaccination coverage data.
- Smoking & Tobacco Use: Provides extensive information about tobacco use in the United States.
- CDC Digital Media Metrics: Offers metrics related to CDC's digital presence.
- Kaggle: An online community for data scientists and machine learners, Kaggle offers extensive datasets and competitions in various healthcare domains. From patient-level medical records to disease progression analysis, Kaggle's datasets provide a rich playground for aspiring data scientists and AI researchers in the field of healthcare.
Collaboration with Medical Care Providers
Collaboration with healthcare providers offers a treasure trove of valuable data for developing and improving AI-powered digital health products. Here's a closer look at the process, along with the crucial considerations for responsible data collection:
Partnerships with hospitals, clinics, or other care providers can be invaluable. They can offer access to a broad range of current, real-world data.
However, this approach requires diligent adherence to regulations and maintaining patient confidentiality. The data must be carefully de-identified to protect patient privacy. Collaborating entities such as Redox must have data usage agreements stipulating how the data will be used, stored, and secured. Successful collaboration hinges on building trust with healthcare providers. Transparency about data usage, data security practices, and the potential benefits of the AI product are key.
Strategies for effective collaboration with medical providers:
- Identify the right partners: Seek collaborators whose patient population aligns with the target audience for your AI product.
- Clearly define goals: Clearly articulate the specific data needs and how the data will be used to develop the AI product.
- Data security expertise: Ensure your team has expertise in data security and compliance with relevant regulations.
- Open communication: Maintain open and transparent communication with partners throughout the collaboration process.
- Focus on benefits: Clearly communicate the potential benefits of the AI product for improving patient care and healthcare delivery.
By carefully navigating these considerations, collaboration with medical care providers can be a powerful tool for sourcing high-quality data to fuel innovation in the field of digital health. Remember, responsible data collection and patient privacy are paramount for building trust and ensuring the ethical development of AI-powered solutions in healthcare.
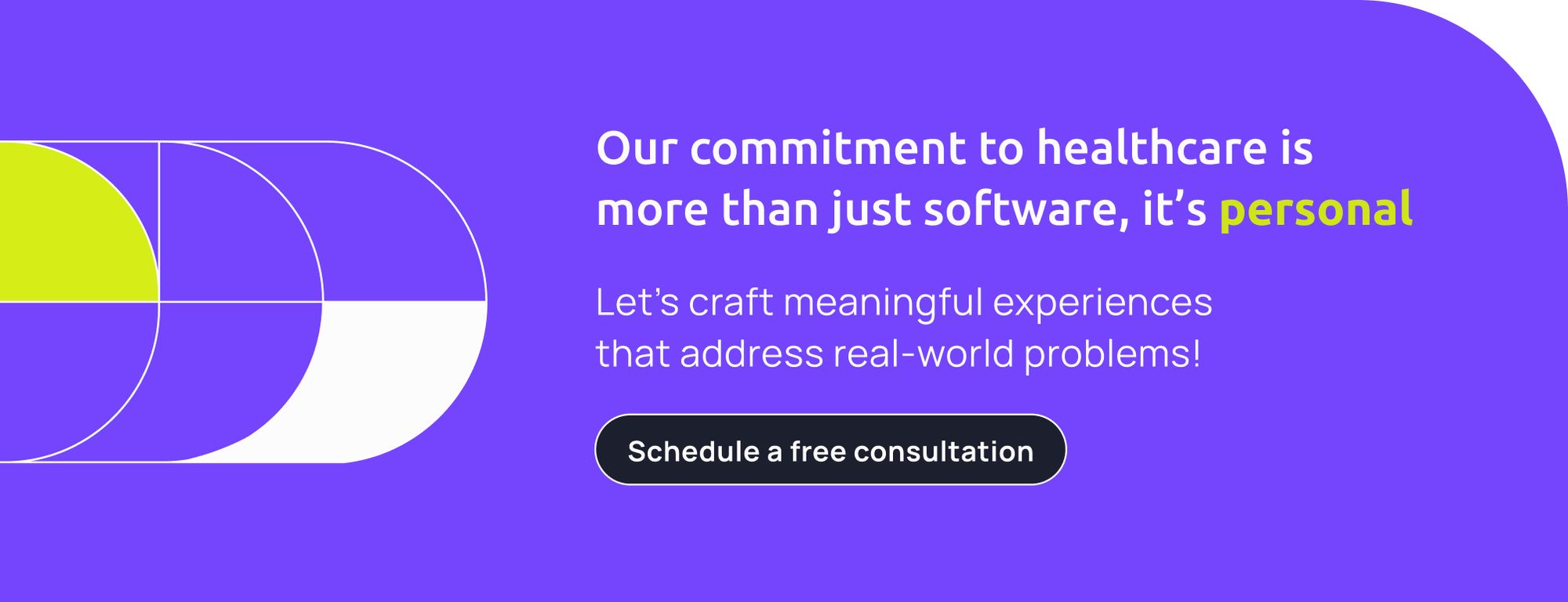
IoT and Mobile Devices
The proliferation of wearables, mobile health apps, and IoT devices in healthcare opens up new sources of continuous, real-time patient data., providing valuable insights into health and well-being.
The primary challenge here is patient consent and privacy. Users must be clearly informed about the collected data and how it will be used and secured. Given the personal nature of such data, stringent security measures are necessary to prevent data breaches.
Strategies for responsible data collection from IoT and mobile devices:
- Transparency and user control: Provide clear and concise explanations about data collection practices within mobile apps and wearables. Offer users granular control over what data is collected and how it's used.
- Focus on user benefits: Clearly communicate the potential benefits of data collection for improving patient care and developing personalized health solutions.
- Secure data storage and processing: Implement robust security measures to protect patient data, following industry best practices and relevant regulations.
- Standardization efforts: Support initiatives to develop standardized data formats for healthcare devices and apps to facilitate easier data sharing and analysis.
Synthetic Data
Synthetic data generation stands as one of the most cutting-edge methods for acquiring data in healthcare, pushing the boundaries of what's possible. Unlike traditional methods, it leverages the power of Generative AI (GAI) to create entirely new datasets that resemble real-world patient data but without privacy concerns or limitations.
This algorithmically generated data that mimics real-world data can be an effective solution when real patient data is limited or privacy concerns are high. It enables the simulation of various scenarios without risking patient privacy.
However, it's crucial to ensure that synthetic data accurately mirrors the characteristics of real-world data to avoid introducing bias or inaccuracies in the AI models. Rigorous validation of synthetic data against actual data is necessary to ensure its quality and usefulness.
Some examples of synthetic data services are:
Considerations for responsible synthetic data generation:
- Technical Expertise: Generative AI is a tool that demands knowledge to use. Building and managing these models demands a deep understanding of machine learning and AI concepts.
- Data Validation: Just like comparing a new painting to the original masterpiece, synthetic data needs rigorous validation. Researchers must ensure the generated data accurately reflects real-world characteristics. Statistical properties and clinical relevance need to be meticulously checked.
- Explainability and Transparency: Understanding how AI artists create synthetic data is crucial. Explainability techniques help ensure the data is reliable and ethically sound. We need to be able to see the logic behind the AI's brushstrokes.
Data Purchased from Brokers
Data brokers aggregate data from various sources, which can provide an additional or alternative data source. They often have datasets from different geographical regions and demographics, providing a wider scope.
However, the use of brokered data carries substantial regulatory and ethical implications. The origin of the data and the consent procedures must be clear. Data security measures employed by the broker should meet high standards to ensure compliance with regulations such as HIPAA and the General Data Protection Regulation (GDPR).
Strategies for mitigating risks while buying data from brokers:
- Scrutinize the broker: Before purchasing data, thoroughly vet the data broker. Investigate their data sourcing practices, security measures, and compliance with relevant regulations.
- Focus on transparency: Seek data brokers who can provide clear documentation on the origin of the data, the consent procedures followed, and the data anonymization process.
- Data minimization: Only purchase the specific data points strictly necessary for your AI development project. Avoid acquiring vast datasets containing irrelevant information.
- Supplement with other sources: Combine data from brokers with information obtained through collaborations with healthcare providers or anonymized patient surveys. This triangulation approach can help enhance data quality and address potential biases.
Conclusion
Sourcing data for AI in digital health is not a one-size-fits-all process. The right approach depends on the specific requirements of the AI application, regulatory landscape, and ethical considerations. Balancing the need for comprehensive data with patient privacy and regulatory compliance is key. The strategies discussed here offer diverse pathways to acquiring data, each with its strengths and challenges.
Tackling this complex space is a necessary journey to harness the potential of AI in delivering more efficient, effective, and personalized healthcare. Remember, responsible data collection and patient privacy are paramount for building trust and ensuring the ethical development of AI-powered solutions in healthcare.